Today in Edworking News we want to talk about Testing Generative AI for Circuit Board Design
TLDR:
We test LLMs to figure out how helpful they are for designing a circuit board. We focus on utility of frontier models (GPT4o, Claude 3 Opus, Gemini 1.5) across a set of design tasks, to find where they are and are not useful. They look pretty good for building skills, writing code, and getting useful data out of datasheets.
Introduction
Can an AI-powered chatbot help with a task as precise as circuit board design? These LLMs (Large Language Models) are famous for hallucinating details, and missing a single important detail can sink a design. Determinism is hard but super important for electronics design! Today, several shallow product offerings are making AI for electronics design look mostly like hype. But we believe there is real utility to be found here, if we can take a better approach.
In this article, we set LLMs to challenging tasks that expert human circuit board designers handle daily. We're not looking for basic help, rather pushing on what it takes to help an expert do their job better.
Models Tested
Gemini 1.5 Pro from Google
GPT-4o from OpenAI
Claude 3 Opus from Anthropic
We explore prompting strategies to get the best performance out of each model and test their capabilities in building skills, writing code, and extracting useful data from datasheets.
Asking Stupid Questions
There's a lot to know in circuit board design, and nobody has mastered every domain. Asking an LLM stupid questions is a great way to learn. For example, an RF engineer might not know much about supply chain or power supply design. To simulate someone new to a domain, basic questions without expert vocabulary help evaluate the LLM’s utility.
Example Query
What is the delay per unit length of a trace on a circuit board?
Claude 3 Opus excelled by bringing in relevant concepts and got the answer right while pointing out critical nuances.
Google Gemini 1.5 performed poorly, likely due to incorporating low-quality internet sources.
Finding Parts
An experienced engineer often knows the approximate size and cost of components. We tested LLMs by asking them to suggest parts for a communications layer for a motor driver using 100M optical Ethernet.
Prompts and Responses
Despite using detailed specifications and prompt engineering, all models performed poorly here. They struggled to suggest the right parts and failed to account for necessary nuances.
Representative Conclusion from Gemini 1.5
Example Part Selection:
- Optical Connectors: LC Duplex connectors (Amphenol or similar)
- Optical Transceivers: 100Base-FX SFP transceivers with industrial temperature ratings
- Ethernet Networking Device: Microchip LAN8742A or Texas Instruments DP83848
Grading: All models missed the requirement for a three-port Ethernet switch and suggested inappropriate transceivers for a compact robot joint.
Parsing Datasheets
Critical design data is often stored in PDF datasheets. We tested three ways of pulling information:
Copy/paste from PDF and prompt
Capture an image and have the LLM interpret
Upload entire PDF
Example Case
Using the Nordic nRF5340 WLCSP with an 820-page datasheet, our best method was loading the entire datasheet and querying interactively.
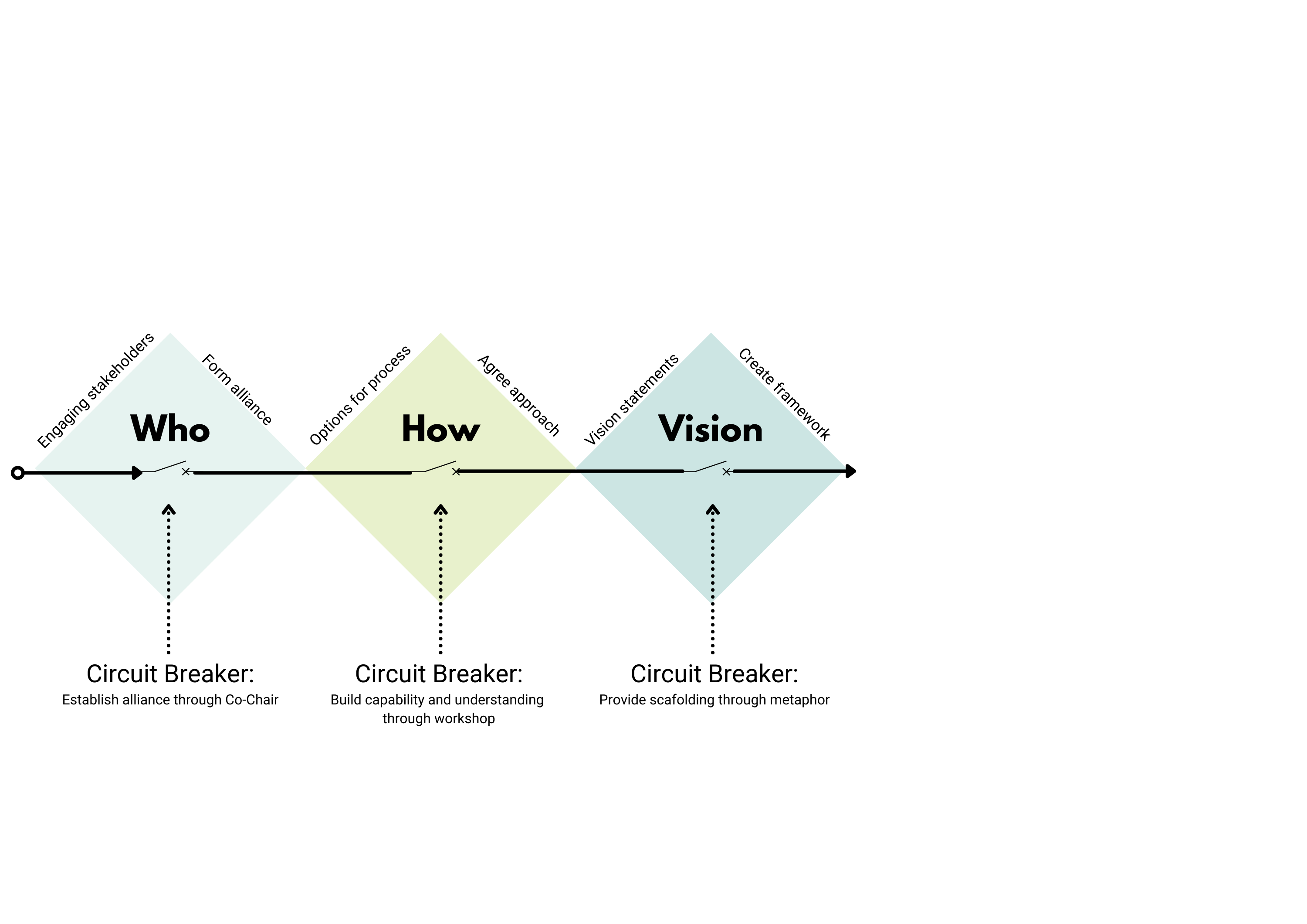
Image Description: An example of a circuit board designed using AI assistance
Designing Circuits
LLMs can understand schematics well enough to turn them into netlists. Can they design a circuit itself? We tested an analog circuit design task.
Task: Design a microphone pre-amplifier
The prompt included requirements for biasing an electret microphone and creating a single-ended signal to drive an ADC.
Claude 3 Opus provided the best layout proposals but made some fundamental errors.
LLMs tend to attach capacitors across positive and negative pins indiscriminately.
Higher-Level Code
In practical design, higher-level functions are used. LLMs did better at inventing and using higher-level functions.
Example
Gemini 1.5 invented reasonable APIs, but still, misses surfaced in netlist generation.
Conclusion
Circuit board design requires precision, and unsupervised AI techniques face data challenges. Design context and meaning aren't encapsulated purely in textual data. Our experiments show that LLMs are useful for specific tasks like extracting and transforming information, but struggle with generating accurate, complex designs.
Edworking is the best and smartest decision for SMEs and startups to be more productive. Edworking is a FREE superapp of productivity that includes all you need for work powered by AI in the same superapp, connecting Task Management, Docs, Chat, Videocall, and File Management. Save money today by not paying for Slack, Trello, Dropbox, Zoom, and Notion.
Remember these 3 key ideas for your startup:
Learning New Domains: Utilizing chatbots like Claude 3 Opus can help quickly cover new areas in circuit board design, emphasizing critical details.
Data Extraction: Gemini 1.5 large context window is very effective for pulling detailed component data from dense datasheets, simplifying otherwise tedious tasks.
Higher-Level Code Generation: Employing LLMs to generate higher-level code rather than raw netlists can improve accuracy in complex designs, saving time and reducing errors.
For more details, see the original source.