Last week, I was helping a friend of mine to get one of his new apps off the ground. I can’t speak much about it at the moment, other than like most apps nowadays, it has some AI sprinkled over it. Ok, maybe a bit more than just a bit – depends on the way you look at it, I suppose. There is a Retrieval-augmented generation (RAG) hiding somewhere in most of the AI apps. RAG is still all the RAGe – it even has its own Wikipedia page now! I’m not sure if anyone is tracking how fast a term reaches the point where it gets its own Wiki page, but RAG must be somewhere near the top of the charts. I find it quite intriguing that most of the successful AI apps are basically clever semantic search apps. Google search got somewhat unbundled at last, which kind of makes me think whether their not unleashing all the LLM tech way earlier was behind all of this. But I digress. The app my friend has been building for the past couple of weeks deals with a lot of e-commerce data: descriptions of different items, invoices, reviews, etc. The problem he was facing was that the RAG wasn’t working particularly well for some queries, while it worked very well for others.
Understanding Tokenization in AI Apps
One of the things I noticed over the past year is how a lot of developers who are used to developing in the traditional (deterministic) space fail to change the way they should think about problems in the statistical space which is ultimately what LLM apps are. Statistics is more “chaotic” and abides by different rules than the “traditional” computer science algorithms. Look, I get it, it’s still maths, but it’s often a very different kind of maths. What I usually see is folks thinking about LLMs as tools you can feed anything and get back gold, but in reality, when you do that, you usually encounter “Garbage In, Garbage Out” reality. Which was almost the case in the curious case the friend of mine was dealing with.
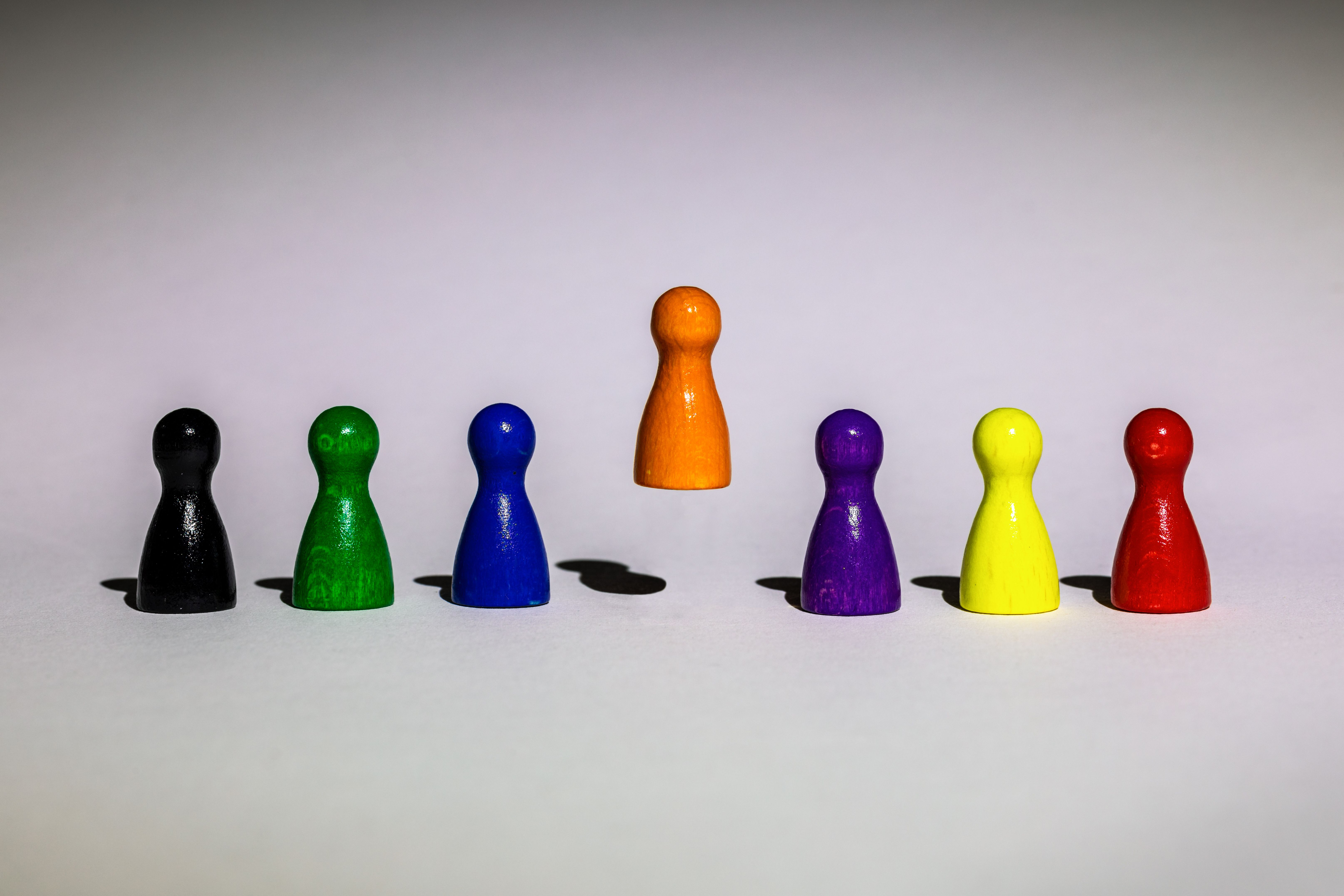
Image: Visual representation of the tokenization process.
The first thing I do when dealing with these types of problems is getting myself familiar with the input data. You need to understand those before you can do anything meaningful with it. In this case, the input data was both the raw text that was indexed and stored in the vector databases as well as the user queries used in the retrieval. Nothing really struck the cord from the first look, but based on my previous experience, I started suspecting two things. Actually, more than two, but more on that later: Chunking is more or less a fixable problem with some clever techniques: these are pretty well documented around the internet; besides, chunking is a moving target and will only get you so far if your text tokens are garbage.
The Importance of Tokenization
In this post, I want to focus on tokenization because I feel like it’s one of those things that is somewhat understood from a high-level point of view, but the deeper you dig in, the more gaps in your knowledge you will discover and from my experience it’s often those gaps that often make or break AI apps. I’m hoping this post will demonstrate some practical examples that will convince you why you should pay attention to tokenizers.
Tokenization is the process during which a piece of text is broken down into smaller pieces, tokens, by a tokenizer. These tokens are then assigned integer values (a.k.a. token IDs) which uniquely identify the tokens within the tokenizer vocabulary. The tokenizer vocabulary is a set of all possible tokens used in the tokenizer training: yes, the tokenizers are trained (I feel the term is a bit overloaded because the tokenizer training is different from neural network training). You can train your own tokenizer and restrict its token space by various parameters, including the size of the vocabulary.
Now, if you started asking yourself what happens if any of the tokens in your text do not exist in the tokenizer’s vocabulary of the LLM you are trying to use, then you probably understand where this is headed now: usually a world of pain and hurt for many. Do not panic! A lot of the large LLM vocabularies are pretty huge (30k-300k tokens large). There are different types of tokenizers used by different LLMs, and it’s usually a good idea to be aware of which one is used by the LLM you are trying to use in your app.
Embeddings and Their Role
Tokenizers on their own are kinda….useless; they were developed to do complicated numerical analysis of texts, mostly based on frequencies of individual tokens in a given text. What we need is context. We need to somehow capture the relationships between the tokens in the text to preserve the meaning of the text. There is a better tool for preserving contextual meaning in the text: embeddings i.e. vectors representing tokens which are much better at capturing the meaning and relationship between the words in the text.
Embeddings are a byproduct of transformer training and are actually trained on the heaps of tokenized texts. It gets better: embeddings are what is actually fed as the input to LLMs when we ask it to generate text. The LLMs consist of two main components: encoder and decoder. Both the encoder and decoder accept embeddings as their input. Furthermore, the output of the encoder is also embeddings which are then passed into the decoder’s cross-attention head which plays a fundamental role in generating (predicting) tokens in the decoder’s output.
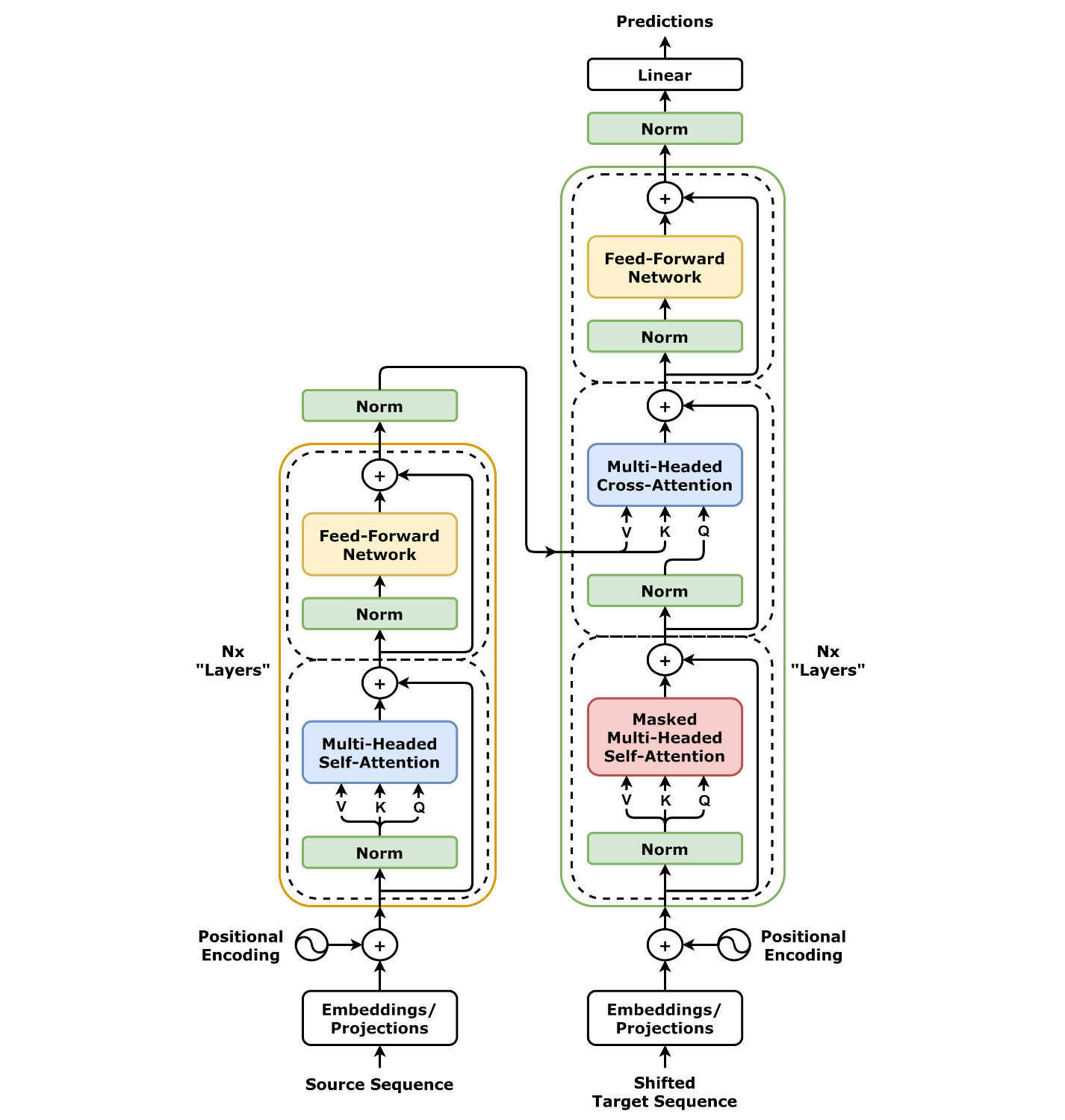
Image: Diagram of a typical transformer architecture.
Here’s what a transformer architecture looks like: So in your RAG pipeline, your text is first tokenized, then embedded, then fed into the transformer where the attention does its magic to make things work well. Earlier I said the token IDs are essentially indexes into the tokenizer vocabulary. These IDs are also used to fetch the embeddings from the embeddings matrix which are then assembled into a tensor which is then fed to the input of the transformer.
Conclusion
I hope this post gave you a better idea about how tokenizers may influence your RAG apps and why you should pay at least some attention to them. More importantly, I hope you now understand that garbage-in garbage-out will not always pay the dividends you might expect in your agentic applications. A little bit of cleaning of input text (you noticed the effect some empty space characters had on embeddings) might go a long way: standardize the format your dates so they’re consistent throughout your embeddings; remove trailing spaces wherever you can - you saw the effect they had on the embeddings; the same goes for any other numerical data like prices in different currencies, etc.
Remember these 3 key ideas for your startup:
Understand the Importance of Tokenization: Tokenization is crucial for AI apps, especially those using LLMs. It breaks down text into manageable tokens, which are then used for analysis and processing. Understanding how tokenization works can help improve the accuracy and efficiency of your AI applications. For more insights on managing AI projects, check out this quick guide to strategy portfolio management.
Leverage Embeddings for Contextual Understanding: Embeddings capture the relationships between tokens, preserving the meaning of the text. They are essential for providing context in AI models, ensuring that the generated outputs are meaningful and relevant. Learn more about how to effectively assign tasks to team members to improve your team's productivity.
Optimize Your Input Data: Clean and standardize your input data to avoid the "Garbage In, Garbage Out" scenario. Pay attention to details like date formats, currency symbols, and spacing to ensure your AI models perform optimally.
Edworking is the best and smartest decision for SMEs and startups to be more productive. Edworking is a FREE superapp of productivity that includes all you need for work powered by AI in the same superapp, connecting Task Management, Docs, Chat, Videocall, and File Management. Save money today by not paying for Slack, Trello, Dropbox, Zoom, and Notion. For more details, see the original source.