Diffusion Forcing enjoys key strengths of both next-token autoregressive models and full-sequence diffusion models. By training Diffusion Forcing once, one can flexibly control its behavior at sampling time to simultaneously perform flexible and compositional generation like next-token models and perform sequence-level guidance like full-sequence diffusion models. Diffusion Forcing achieves this by training sequence diffusion but allowing each token to have a different noise level. One can view noises in diffusion as varying levels of masking and establish a unified view: full-sequence diffusion denoises all frames at once with the same noise level, while next-token prediction denoises the next frame at a time with zero noise in its past tokens. As a result, one can use different noise levels across a sequence at sampling time to achieve flexible behaviors such as stabilizing auto-regressive rollout, guidance over long horizons, or planning with causal uncertainty.
Video Prediction
We provide a list of synthesized videos directly generated by models (without VAE/superresolution). The below results are sampled without cherry-picking. Video Prediction by Diffusion Forcing (ours) and baselines in DMLab dataset (0.25x speed). Teacher forcing easily blows up while causal full-sequence diffusion models suffer from serious consistency issues. Diffusion Forcing can achieve stable and consistent video prediction. PNG visualizations are provided below to reflect the original quality of generated samples.
Video Prediction by Diffusion Forcing (ours) and baselines in Minecraft dataset (0.5x speed). Teacher forcing easily blows up while causal full-sequence diffusion models suffer from serious consistency issues. Diffusion Forcing can achieve stable and consistent video prediction. PNG visualizations are provided below to reflect the original quality of generated samples.
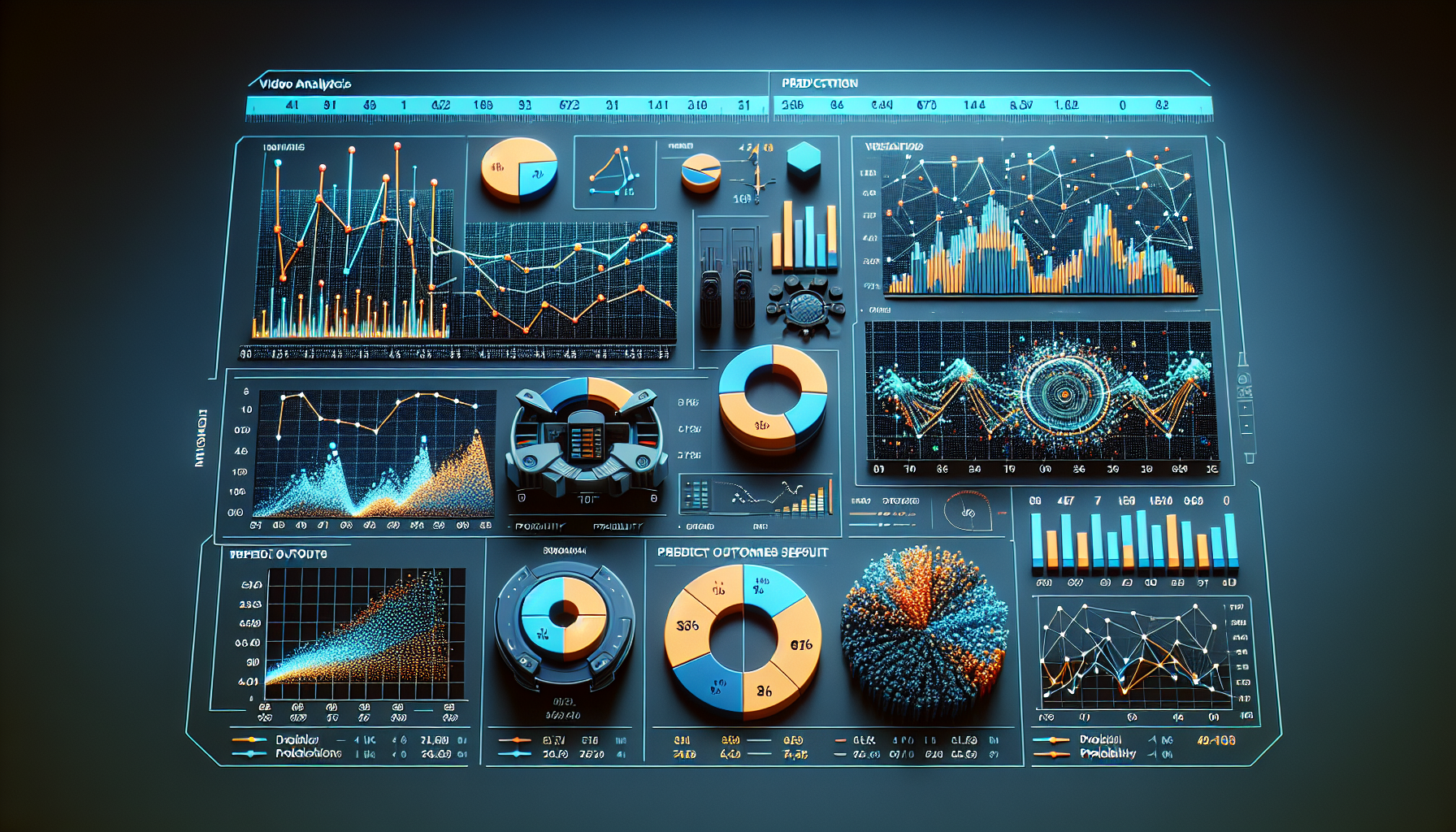
Stabilizing Infinite Rollout without Sliding Window
In addition, one can rollout much longer videos with our method than the maximum sequence length it's trained on. Remarkably, we can do this without Sliding Window. That is, we rollout RNN without ever resetting the latent z to the initial latent z0, showing the stabilization effect of Diffusion Forcing thanks to its stabilization effect. Videos are compressed for loading speed. The results are sampled without cherry-picking. Quality of the video is decreased due to mp4 compression of long videos! We provide PNG visualizations below to reflect the original quality of generated samples longer than the training horizon. Diffusion Forcing (ours) trained on 36 frames can rollout for 2000 frames or more on the DMLab dataset, without sliding window thanks to its stabilization effect. Videos are compressed for loading speed. Original dataset resolution is 64x64. Quality of the video is decreased due to mp4 compression of long videos! We provide PNG visualizations below to reflect the original quality of generated samples longer than the training horizon.
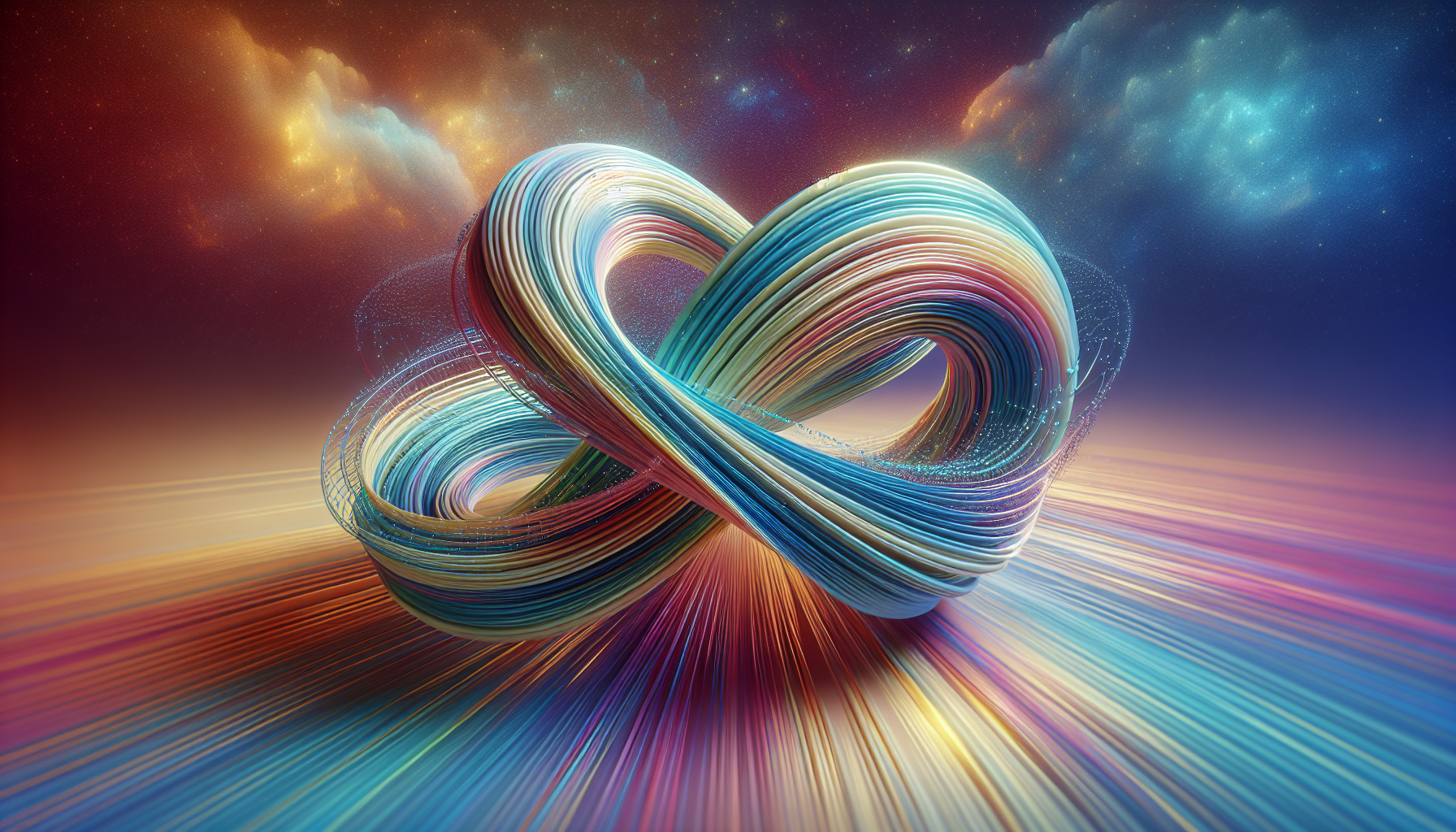
Diffusion Forcing (ours) trained on 72 frames rollouts for 2000 frames or more on Minecraft dataset without blowing up, without sliding window. Original dataset resolution is 128x128. In certain scenarios, the agent will get stuck in front of two-block-high dirt or stone blocks until it switches direction, which is an intrinsic issue of the dataset collection.
Diffusion Planning
Similar to prior works like Diffuser, we can use test-time guidance to make our diffusion sequence a planner. However, we explicitly model the causal relationship by defining each token as [a_t, o_{t+1}]. By doing so, we have a belief over the action to take and the observation it’s leading to, but can also update this belief to posterior estimation when a new observation is made after the action is taken. Visualization of the diffusion planning process of Diffusion Forcing as a decision-making framework. To model the causal uncertainty of the future, diffusion forcing's plan can have near future at lower noise level while having far future at higher noise level.
Long Horizon Imitation Learning
Many real-world tasks are not Markovian and require long-horizon memory to accomplish. In our real robot task, a robot arm is asked to swap the slots of two fruits using a third slot. Since the fruits are input in random slots at the beginning, one cannot determine the next steps from a single observation without knowledge of the initial placement of the fruits. We simply remove guidance from the planning experiments and jointly diffuse action-observation sequences to perform feedback control. The above video shows multiple continuous successes before a failure happens. One can observe that the robot is able to accomplish the task even when the fruit location is randomized by the previous run. On the other hand, we tried SOTA imitation learning techniques, Diffusion Forcing. But it cannot perform the task due to non-Markovianess. In addition, diffusion forcing can be prompted to treat incoming observation as noisy ones to be robust to unseen distractions at test time. In the video above, we illustrate our distraction method of randomly throwing a shopping bag into the field of view.
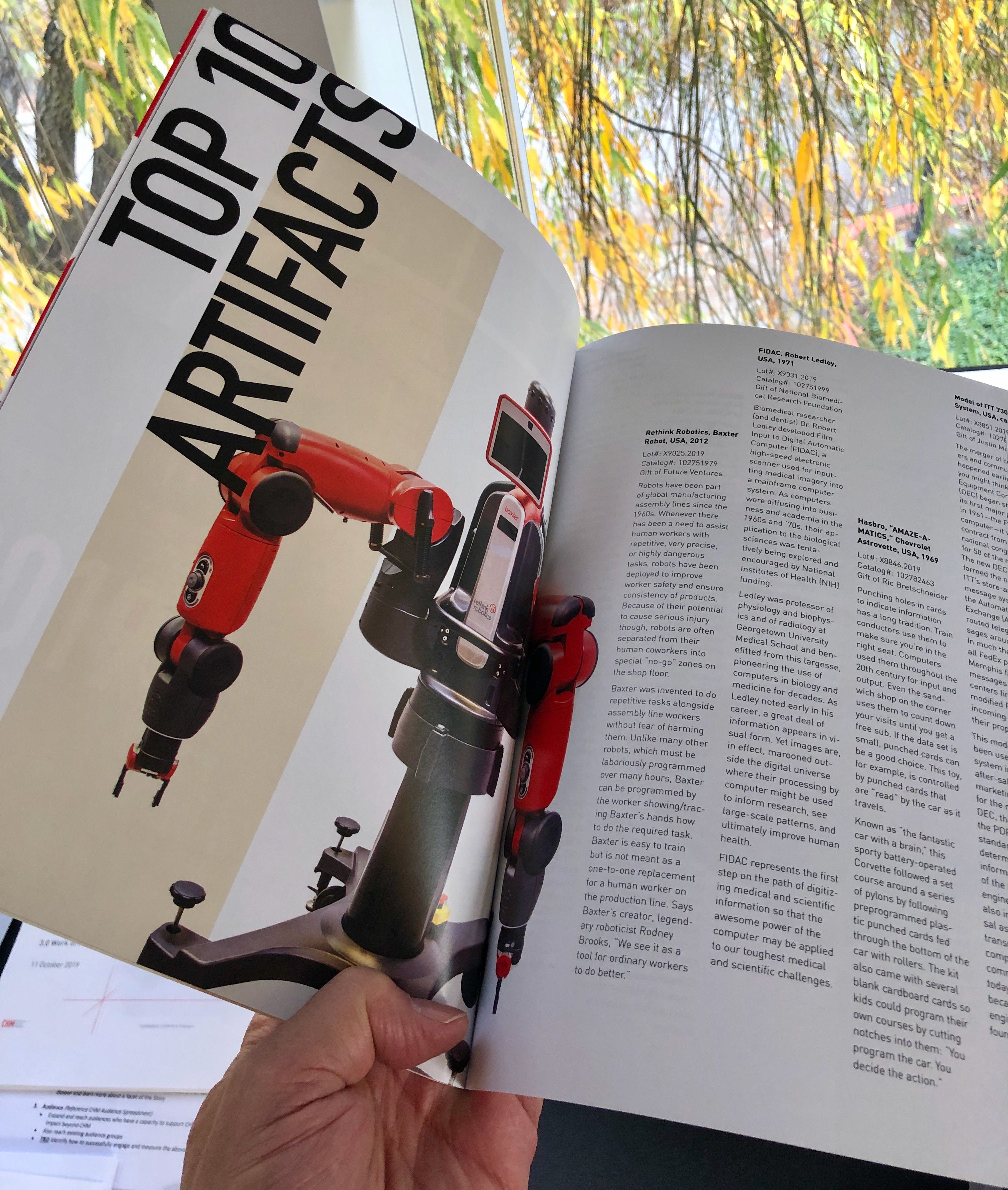
Remember these 3 key ideas for your startup:
Flexible Behavior Control: Diffusion Forcing allows for flexible and compositional generation as well as sequence-level guidance. This can be instrumental for startups needing adaptable AI solutions. Explore more on how to brand yourself as a remote company.
Stabilizing Long-term Predictions: Whether it's video generation or robotic tasks, Diffusion Forcing ensures stability and consistency over long sequences, which can be critical for maintaining reliability in tech products. Learn more about project monitoring and how to track and control projects.
Enhanced Planning and Decision-making: Utilizing Diffusion Forcing for long-horizon imitation learning and planning enhances decision-making, a crucial element in industries like robotics and AI-driven automation. For more information, check out our comprehensive guide to agile transformation.
Edworking is the best and smartest decision for SMEs and startups to be more productive. Edworking is a FREE superapp of productivity that includes all you need for work powered by AI in the same superapp, connecting Task Management, Docs, Chat, Videocall, and File Management. Save money today by not paying for Slack, Trello, Dropbox, Zoom, and Notion.
For more details, see the original source.